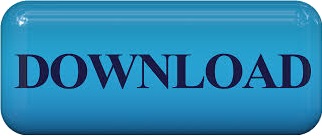
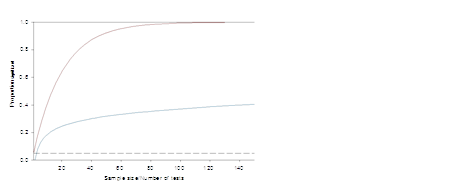
We introduce a new framework for two‐sample multiple testing by incorporating a carefully constructed auxiliary variable in inference to improve the power. However, this data reduction step could cause significant loss of information and thus lead to suboptimal testing procedures. The conventional practice first reduces the original observations to a vector of p‐values and then chooses a cut‐off to adjust for multiplicity. Two‐sample multiple testing has a wide range of applications. Not accounting for measurement variability can be shown to not only result in unnecessary on-site deferrals but also results in donations by donors that can be shown not to comply with the legally required minimum Hb levels. Contrarily, it appeared that 460 donors (0.10%) made 704 donations (0.06%) whilst not complying with donor eligibility criteria. It was found that 92% of all deferrals were unnecessary as Hb levels were within the range of expected measurement variability. Historical data from 439,376 new donors with a deferral rate of 5.3% were analyzed by applying the new donor deferral algorithm. The algorithm was tested on a cohort of new Dutch donors that started between 20 to evaluate its impact on the donor deferral rate. This information is incorporated in a new algorithm for donor deferral where the mean hemoglobin level of a donor is used to determine both donor eligibility and the deviance of individual measurement outcomes. Repeated hemoglobin measurements obtained at donor intake were used to estimate the variability in measurement outcomes (measurement variability). Despite many efforts, the main cause of donor deferral-the variability in hemoglobin measurement outcomes-remains largely unaddressed. Blood banks continuously strive to reduce deferral rates as these imply an immediate loss of products, a waste of materials, a waste of staff and donor time, and potential loss of donors. On-site deferral for low hemoglobin (Hb) is common in most countries and deferral rates commonly vary between 1% and 20%. We also provide simulation results comparing different online testing algorithms and an up-to-date overview of the many methodological extensions that have been proposed. In this paper, we present a comprehensive exposition of the literature on online error rate control, with a review of key theory as well as a focus on applied examples. In this framework, hypotheses arrive in a stream, and at each time point the analyst decides whether to reject the current hypothesis based both on the evidence against it, and on the previous rejection decisions.
#False discovery rate sequential testing how to#
To address these challenges, a new field of methodology has developed over the past 15 years showing how to control error rates for online multiple hypothesis testing. However, traditional procedures that control the FDR, such as the Benjamini-Hochberg procedure, assume that all p-values are available to be tested at a single time point. In many biomedical and technological applications, an additional complexity is that hypotheses are tested in an online manner, one-by-one over time. Modern data analysis frequently involves large-scale hypothesis testing, which naturally gives rise to the problem of maintaining control of a suitable type I error rate, such as the false discovery rate (FDR). We present theory and numerical results to show that SAST is asymptotically valid for online FDR control and achieves substantial power gain over existing online testing rules. SAST captures time varying structures of the data stream, learns the optimal threshold adaptively in an ongoing manner and optimizes the alpha-wealth allocation across different time periods. A key element in our proposal is a new alpha–investing algorithm that precisely characterizes the gains and losses in sequential decision making. This work develops a new class of structure–adaptive sequential testing (SAST) rules for online false discovery rate (FDR) control. Moreover, the online decision–making process may come to a halt when the total error budget, or alpha–wealth, is exhausted. Conventional simultaneous testing rules are no longer applicable due to the more stringent error constraints and absence of future data. The error rate is required to be controlled at all decision points. Consider the online testing of a stream of hypotheses where a real–time decision must be made before the next data point arrives.
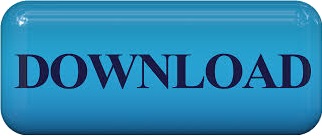